How do we make AI trustworthy?
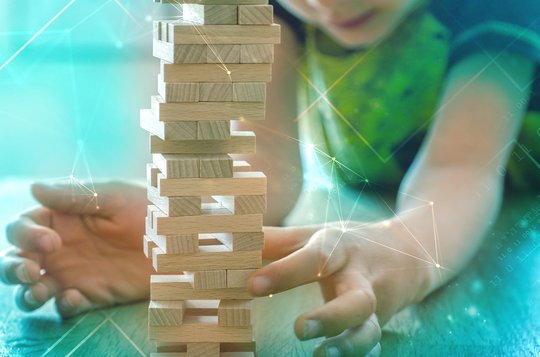
Due to the nature of AI-based systems, additional AI-specific risks arise in projects of this type in addition to the classic product and project risks, such as the AI-based system making incorrect decisions due to incorrect or insufficiently representative training data. Furthermore, incorrectly selected training data can lead to phenomena such as bias, overfitting, underfitting or other quality problems as well as likewise incorrect decisions being learned by the AI.
In addition, a certain proportion of people, in this specific case a proportion of users or affected persons, may not accept decisions made by autonomous systems or even artificial intelligence. It is therefore possible that an AI-based system objectively fulfills all requirements or even shows significantly better results than the use of conventional systems or human decision-makers, but that it is not purchased or used because the users do not have confidence in the system.
This is why new quality characteristics are emerging in the artificial intelligence environment, such as "trustworthiness", which expresses the degree of trust a user has, or "explainability", which provides AI users with a situational and comprehensible explanatory model, e.g. in order to be able to understand a decision that has been made. Depending on the context, other quality characteristics can also play a role in the use of AI-supported systems. Here, too, the corresponding quality characteristics must be examined and their fulfillment must be ensured with suitable quality assurance.
imbus can advise you on the selection of appropriate quality characteristics and metrics and their testing, as well as checking for compliance with standards and legal regulations, and support you with appropriate QA measures and tests..
Ihr Ansprechpartner bei imbus
Mr. Tilo Linz
mail: tilo.linz@imbus.de
phone: 09131 7518-210
fax: +49 9131 / 7518-50